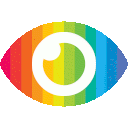
1. Connected automated vehicles (CAVs) provide opportunities for optimal intersection control by integrating connected vehicle (CV) and automated vehicle (AV) technologies. CVs can communicate with each other and with infrastructure to exchange real-time traffic data, while AV technologies enable precise trajectory planning and control.
2. Previous studies have explored the integration of CV data into traffic signal control, as well as fully controlling CAV trajectories based on real-time signal phasing and traffic conditions. These studies have used various optimization algorithms, such as dynamic programming and model predictive control, to reduce delay and improve traffic efficiency.
3. The proposed DRL-TP3 framework combines deep reinforcement learning (DRL) with TP3 (trajectory planning with piecewise polynomials) to simultaneously optimize signal control and smooth vehicle trajectories in mixed traffic environments. By using a DDPG model trained through TD learning, the framework can efficiently update signal timing plans with finer resolution and control vehicular trajectories in real time. This approach overcomes the computational burden of traditional methods like dynamic programming and enables joint control in large-scale scenarios.
对于上述文章,我无法提供详细的批判性分析,因为只提供了文章的一部分内容。请提供完整的文章或更多相关信息,以便我能够进行全面的分析和评价。