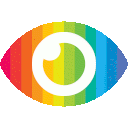
1. The combination of machine learning and untargeted metabolomics was used to identify the lipid metabolism-related gene CH25H as a potential biomarker in asthma.
2. CH25H was found to be highly expressed in two mouse models of asthma, suggesting its involvement in the disease.
3. A lipid metabolism score constructed from five genes, including CH25H, was able to effectively distinguish different subtypes of asthma.
The article titled "The combination of machine learning and untargeted metabolomics identifies the lipid metabolism-related gene CH25H as a potential biomarker in asthma" explores the role of lipid metabolism in asthma and identifies CH25H as a potential biomarker for the disease. While the study utilizes machine learning algorithms and untargeted metabolomics to analyze data from mouse-derived datasets and a human dataset, there are several potential biases and limitations that need to be considered.
One potential bias is the selection of datasets from the GEO database. The authors do not provide a clear rationale for selecting these specific datasets, which may introduce selection bias. Additionally, it is unclear how representative these datasets are of the broader population of individuals with asthma.
Another limitation is the use of machine learning algorithms to identify core genes. While these algorithms can be useful for identifying patterns in large datasets, they are not without their limitations. The choice of algorithms used in this study may introduce bias or overlook important genes that could be relevant to lipid metabolism in asthma.
Furthermore, the study relies on non-targeted metabolomics to identify differentially expressed metabolites in asthmatic mice. While this approach can provide valuable insights into metabolic changes associated with asthma, it is important to note that non-targeted metabolomics has its limitations. It may miss certain metabolites or fail to accurately quantify their levels.
The article also claims that CH25H is a core lipid metabolism gene in asthma based on its expression levels in two mouse models of asthma. However, it is unclear how well these findings translate to humans and whether CH25H expression levels can serve as a reliable biomarker for asthma diagnosis.
Additionally, while the article mentions Western blot confirmation of altered expression levels of core genes in stimulated and challenged mice, it does not provide detailed results or statistical analysis of these findings. This lack of information makes it difficult to assess the robustness and significance of these results.
Moreover, there is a lack of discussion on potential confounding factors or alternative explanations for the observed changes in lipid metabolism and metabolite expression. Asthma is a complex disease with multiple contributing factors, and it is important to consider other variables that may influence lipid metabolism and metabolite levels.
The article also does not discuss the potential risks or limitations of using CH25H as a biomarker for asthma diagnosis. It is important to consider the specificity and sensitivity of this biomarker, as well as any potential false positives or false negatives that may arise.
Overall, while the study provides interesting insights into the role of lipid metabolism in asthma and identifies CH25H as a potential biomarker, there are several biases, limitations, and missing points of consideration that need to be addressed. Further research is needed to validate these findings in larger and more diverse populations and to explore the potential clinical implications of using CH25H as a biomarker for asthma.