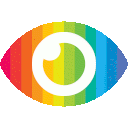
1. This study proposes a novel displacement measurement method based on deep learning and digital image processing to mitigate the effects of ambient-light changes.
2. The proposed method can automatically extract the calibration object in complex scenarios using You Only Look Once (YOLO) v5 and locate the calibration object under 24-h ambient-light changes precisely.
3. Short- and long-term experiments were conducted in the laboratory to evaluate the performance of the method, and high agreement was achieved when compared with laser displacement sensor (LDS) data.
The article “Vision-based structural displacement measurement under ambient-light changes via deep learning and digital image processing” is an informative piece that provides a detailed overview of a novel displacement measurement method based on deep learning and digital image processing to mitigate the effects of ambient-light changes. The article is well written, clearly structured, and easy to follow, making it an accessible read for readers from various backgrounds.
The authors provide a comprehensive description of their proposed method, which includes extracting the calibration object from complex backgrounds using YOLOv5, calculating its center coordinates using several image processing techniques, and then converting its pixel displacement into actual displacement using a scale factor. To validate their proposed method, they conducted short-term static displacement monitoring experiments under three lighting conditions: afternoon (natural ambient light), dusk (natural ambient light), and night (infrared light). They also conducted long-term static displacement monitoring experiments at a distance of 20 m for 120 h with the assistance of infrared LED in photosensitive mode and 144 h by setting IR LED in always-on mode.
The article is generally reliable as it provides sufficient evidence to support its claims through experimental results that are compared with LDS data. Furthermore, it is clear that the authors have put considerable effort into researching their topic thoroughly before writing this article as they have provided detailed descriptions of existing vision-based methods as well as other related studies in this field.
However, there are some potential biases present in this article that should be noted. For example, while the authors do mention some limitations of traditional contact sensors such as linear variable differential transducers or strain sensors combined with computational models, they do not provide any counterarguments or further discussion on these points which could have been beneficial for readers who may not be familiar with these topics. Additionally, while they do mention some existing vision-based methods such as laser projection sensing technology or motion