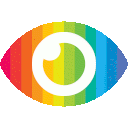
1. Traditional assessment practices have several issues, including being difficult to design and implement, providing only discrete snapshots of performance, being unadapted to participants' backgrounds, adhering to the culture of schooling rather than preparing students for real-world cultures, and assessing skills that are becoming obsolete.
2. Artificial intelligence (AI) approaches can partially address these issues by automating parts of the assessment process, generating assessment tasks, finding appropriate peers to grade work, and automatically scoring student work.
3. However, AI-driven assessments also present unique challenges and require continued research and development to ensure they are effective and equitable.
The article "Assessment in the age of artificial intelligence" argues that traditional assessment practices have several issues, including being difficult for educators to design and implement, only providing discrete snapshots of performance rather than nuanced views of learning, being unadapted to the particular knowledge, skills, and backgrounds of participants, being tailored to the culture of schooling rather than the cultures schooling is designed to prepare students to enter, and assessing skills that humans routinely use computers to perform. The authors review existing AI approaches that partially address these issues but also acknowledge that traditional assessment practices have been successful and valuable for understanding and improving student learning.
The article provides a comprehensive overview of the limitations of traditional assessment practices and how AI can potentially address some of these limitations. However, there are some potential biases in the article. For example, the authors may overstate the limitations of traditional assessment practices while downplaying their benefits. Additionally, they may overemphasize the potential benefits of AI-based assessments without fully exploring their potential risks or drawbacks.
The article also lacks evidence for some claims made. For instance, when discussing how AI can generate assessment tasks, the authors state that "the success of such approaches often relies on the availability of large-scale and relevant datasets used to train those deep neural network models." However, they do not provide any evidence or examples to support this claim.
Furthermore, while the article acknowledges that traditional assessment practices were developed for a reason and have been successful in understanding and improving student learning, it does not fully explore why these practices have persisted despite their limitations. It may be useful to consider factors such as institutional inertia or resistance to change when discussing why traditional assessment practices continue to be used.
Overall, while the article provides a useful overview of how AI can potentially improve assessment practices, it could benefit from more balanced reporting that considers both the benefits and drawbacks of both traditional and AI-based assessments. Additionally, more evidence could be provided for some claims made, and potential risks or drawbacks of AI-based assessments could be explored in more depth.