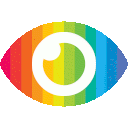
1. The development of smart healthcare technologies, including biomedical sensors and AI-based techniques, has increased interest in smart healthcare systems.
2. ECG heartbeat classification plays a substantial role in smart healthcare systems, as it can help diagnose and treat diseases more intelligently.
3. Machine learning classifiers, such as SVM, RF, kNN, and GBDT, can be optimized using the marine predators optimization algorithm (MPA) to improve the accuracy of ECG arrhythmia classification.
The article titled "ECG Heartbeat Classification Using Machine Learning and Metaheuristic Optimization for Smart Healthcare Systems" discusses the use of machine learning and metaheuristic optimization techniques for classifying ECG heartbeats in smart healthcare systems. While the article provides a comprehensive overview of the topic, there are several potential biases and limitations that should be considered.
One potential bias in the article is the emphasis on the benefits and advantages of smart healthcare systems. The article highlights how smart healthcare systems can process big data at a faster speed with greater accuracy compared to humans. While this may be true to some extent, it fails to acknowledge the limitations and challenges associated with implementing these systems. For example, there may be concerns about data privacy and security, as well as issues related to the reliability and accuracy of the sensors used in these systems.
Another potential bias is the focus on machine learning classifiers over other approaches. The article primarily discusses machine learning classifiers such as SVM, GBDT, RF, and kNN, while only briefly mentioning convolutional neural network (CNN)-based approaches. This bias towards machine learning classifiers may overlook potential benefits or drawbacks of other methods for ECG heartbeat classification.
Additionally, the article lacks a discussion on potential risks or limitations associated with using machine learning algorithms for ECG heartbeat classification. While machine learning algorithms have shown promise in various applications, they are not without their limitations. For example, these algorithms may suffer from overfitting or generalization issues when applied to real-world datasets that differ from the training data. It would have been beneficial for the article to address these risks and provide insights into how they can be mitigated.
Furthermore, there is limited discussion on alternative perspectives or counterarguments to the proposed approach. The article presents machine learning classifiers as an effective solution for ECG heartbeat classification but does not explore potential criticisms or alternative viewpoints. This one-sided reporting limits a comprehensive understanding of the topic and hinders critical analysis.
The article also lacks in-depth discussion and evidence for the claims made. While it mentions previous studies and their results, it does not provide detailed information on the methodologies or datasets used in these studies. This lack of evidence makes it difficult to assess the validity and reliability of the claims made in the article.
In terms of missing points of consideration, the article does not discuss potential ethical implications or societal impacts of implementing smart healthcare systems for ECG heartbeat classification. It would have been valuable to explore issues such as data ownership, consent, and potential biases in algorithmic decision-making.
Overall, while the article provides a comprehensive overview of ECG heartbeat classification using machine learning and metaheuristic optimization techniques, it has several limitations and biases that should be taken into account. The emphasis on the benefits of smart healthcare systems, limited discussion on alternative approaches, lack of evidence for claims made, and missing points of consideration all contribute to a less balanced and critical analysis.