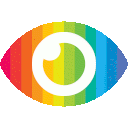
1. PETR is a position embedding transformation for multi-view 3D object detection that encodes the position information of 3D coordinates into image features, producing 3D position-aware features.
2. Object query can perceive the 3D position-aware features and perform end-to-end object detection, achieving state-of-the-art performance on standard nuScenes dataset and ranking 1st place on the benchmark.
3. PETR can serve as a simple yet strong baseline for future research, and its code is available for use.
The article titled "PETR: Position Embedding Transformation for Multi-View 3D Object Detection" presents a new approach to multi-view 3D object detection using position embedding transformation (PETR). The authors claim that PETR encodes the position information of 3D coordinates into image features, producing the 3D position-aware features. Object query can perceive the 3D position-aware features and perform end-to-end object detection. The authors also claim that PETR achieves state-of-the-art performance on standard nuScenes dataset and ranks first place on the benchmark.
The article appears to be well-written and structured, with clear explanations of the proposed method and experimental results. However, there are some potential biases and limitations in this study that need to be considered.
Firstly, the authors do not provide a detailed comparison with other existing methods for multi-view 3D object detection. While they claim that PETR achieves state-of-the-art performance, it is unclear how it compares to other methods in terms of accuracy, efficiency, and robustness. Therefore, it is difficult to assess whether PETR is truly a breakthrough or just an incremental improvement over existing methods.
Secondly, the authors do not discuss the limitations and potential risks associated with their approach. For example, it is unclear how well PETR performs under different lighting conditions or weather conditions. It is also unclear whether PETR can handle occlusions or complex scenes with multiple objects. These limitations could affect the practical applicability of PETR in real-world scenarios.
Thirdly, the article contains some promotional content for their code repository without providing sufficient details about its functionality or usability. While it is good practice to make code available for reproducibility purposes, it would be more informative if the authors provided a detailed description of their codebase and how it can be used by other researchers.
In conclusion, while the article presents an interesting approach to multi-view 3D object detection using PETR, there are some potential biases and limitations that need to be considered. Further research is needed to validate the claims made by the authors and to assess the practical applicability of PETR in real-world scenarios.