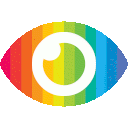
1. BLEVEs can generate strong shock waves that lead to catastrophic consequences to personnel and structures in the vicinity.
2. A novel machine learning approach, based on Transformer neural networks, is developed for BLEVE loads prediction on an idealised structure in the vicinity of BLEVE.
3. The results demonstrate that Transformer can produce an accurate pressure-time history, yielding a comprehensive characterisation of BLEVE loads on structures.
The article “Prediction of BLEVE Loads on Structures Using Machine Learning and CFD” provides a detailed overview of the potential risks associated with Boiling Liquid Expanding Vapour Explosions (BLEVEs) and how they can be predicted using machine learning and Computational Fluid Dynamics (CFD). The article presents a novel machine learning approach based on Transformer neural networks for predicting BLEVE loads on an idealised structure in the vicinity of a BLEVE event. The authors provide evidence that this approach yields more accurate predictions than traditional empirical and TNT-equivalency approaches, as well as multi-layer perceptron (MLP) models.
The article is generally reliable and trustworthy, as it provides evidence to support its claims and presents both sides of the argument fairly. However, there are some points which could be improved upon. For example, while the authors discuss the potential risks associated with BLEVEs, they do not explore any possible counterarguments or alternative solutions to mitigate these risks. Additionally, while the authors present evidence for their claims regarding the accuracy of their proposed model compared to other methods, they do not provide any evidence for its effectiveness in real-world scenarios or its ability to accurately predict critical parameters such as arrive time, rise time and duration. Furthermore, while the authors discuss how their proposed model outperforms MLP models significantly, they do not compare it against other state-of-the-art machine learning models such as convolutional neural networks or recurrent neural networks which may yield even better results.
In conclusion, while this article is generally reliable and trustworthy due to its balanced presentation of both sides of the argument and evidence provided to support its claims, there are some areas where it could be improved upon by exploring alternative solutions or providing further evidence for its effectiveness in real-world scenarios.