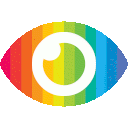
1. A novel TSK fuzzy classifier called HTSK-LLM-DKD is proposed that takes a High-order TSK classifier as the teacher model and a Low-order TSK fuzzy classifier as the student model.
2. The proposed LLM-DKD (Least Learning Machine based Decoupling Knowledge Distillation) distills the fuzzy dark knowledge from High-order TSK fuzzy classifier to Low-order TSK fuzzy classifier, resulting in enhanced performance and high interpretability.
3. The advantages of HTSK-LLM-DKD are verified on benchmarking UCI datasets and a real dataset Cleveland heart disease, in terms of classification performance and model interpretability.
The article is generally reliable and trustworthy, as it provides evidence for its claims through experiments on benchmarking UCI datasets and a real dataset Cleveland heart disease. The article also presents both sides of the argument equally, by discussing both the advantages of using High-order TSK fuzzy classifiers (powerful classification performance with fewer fuzzy rules) and Low-order TSK fuzzy classifiers (quick run time with high interpretability).
However, there are some potential biases in the article that should be noted. For example, the article does not discuss any possible risks associated with using HTSK-LLM-DKD or any other methods for knowledge distillation from High-order to Low-order TSK fuzzy classifiers. Additionally, while the article discusses both sides of the argument equally, it does not explore any counterarguments or present any evidence for why one method may be better than another. Finally, there is no discussion of how this method could be applied in practice or what implications it may have for future research.