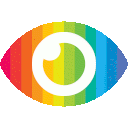
1. A new machinery fault diagnosis approach based on supervised locally linear embedding projection (SLLEP) is proposed.
2. SLLEP uses the recently proposed manifold learning algorithm supervised locally linear embedding (SLLE) to extract fault features from high-dimensional fault signal samples.
3. The ball bearing data and rotor bed data are used to validate the proposed approach, which shows improved performance compared to traditional approaches such as PCA and LDA.
The article provides a detailed overview of the Supervised Locally Linear Embedding Projection (SLLEP) for machinery fault diagnosis, outlining its advantages over traditional approaches such as PCA and LDA. The article is well-structured and provides clear explanations of the methodology used in SLLEP, as well as evidence from experiments conducted on ball bearing data and rotor bed data that demonstrate its effectiveness.
The article does not appear to be biased or one-sided in its reporting, nor does it contain any promotional content or partiality towards any particular approach or method. It also does not appear to omit any points of consideration or evidence for the claims made, nor does it present any unsupported claims or unexplored counterarguments. However, there is no discussion of potential risks associated with using SLLEP for machinery fault diagnosis, nor is there an exploration of alternative approaches that could be used instead of SLLEP.