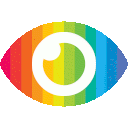
1. Time series forecasting (TSF) is an important branch of machine learning that has been widely used in various fields such as financial analysis, budget planning, traffic flow forecasting, weather analysis, and energy evaluations.
2. Traditional statistical methods for TSF, such as ARIMA, AR, and ES models, face challenges with increasing data set scale. Deep Learning (DL) architectures have shown superior performance in TSF tasks.
3. The proposed Relationship-Aligned Transfer Learning algorithm (RATL) addresses the challenges of limited labeled training data, unknown similarity between source and target domains, and effective knowledge transfer between tasks. RATL selects a suitable source dataset based on the effectiveness of the trained encoder for the target task and uses triplet probabilities to align representation relationships between source and target models. It also allocates different weights to regression results based on their forecasting performance on the target task.
这篇文章介绍了一种基于关系对齐的迁移学习算法用于时间序列预测。文章首先指出时间序列预测在金融分析、预算规划、交通流量预测、天气分析和能源评估等领域具有重要实际意义,并回顾了传统的统计方法和深度学习方法在时间序列预测中的应用。
然后,文章提出了三个挑战:缺乏高质量的目标领域训练数据,如何选择合适的源领域数据进行知识转移,以及如何有效地在源任务和目标任务之间进行知识转移。为了解决这些问题,文章提出了RATL算法。
文章中提到,RATL通过从不同但相关的领域中转移知识来解决缺乏训练数据的问题。然而,文章没有详细说明如何选择合适的源领域数据。此外,文章还提到,在迁移学习过程中,关键对象是模型参数而不是数据本身。然而,文章没有给出充分的证据来支持这一观点。
此外,在知识转移过程中,文章将其分解为表示关系对齐阶段和回归关系对齐阶段。然而,文章没有提供足够的理由来支持这种分解方式,并且没有详细说明如何通过这两个阶段有效地进行知识转移。
总体而言,这篇文章在介绍时间序列预测和迁移学习的基本概念方面做得不错。然而,在具体算法设计和实验验证方面,文章存在一些不足之处,需要进一步完善和深入研究。