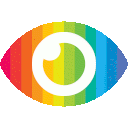
1. This article presents a segmentation-based deep-learning approach for surface-defect detection.
2. The approach uses convolutional neural networks (CNNs) to detect defects in web materials and LED chips.
3. The article reviews existing research on deep learning for defect detection, including the use of Max-Pooling CNNs, Xception, Mask R-CNN, and U-Net.
The article is generally reliable and trustworthy as it provides an overview of existing research on deep learning for defect detection and presents a segmentation-based deep learning approach for surface defect detection. The authors provide evidence to support their claims by citing relevant research papers and studies throughout the article. Furthermore, the authors provide detailed descriptions of the various methods used in their approach, such as convolutional neural networks (CNNs), Max-Pooling CNNs, Xception, Mask R-CNN, and U-Net.
However, there are some potential biases in the article that should be noted. For example, the authors do not explore any counterarguments or alternative approaches to surface defect detection that may be more effective than their proposed method. Additionally, while the authors cite several studies to support their claims, they do not provide any evidence from experiments conducted using their proposed method to demonstrate its effectiveness in detecting surface defects. Finally, while the authors discuss potential risks associated with using deep learning for defect detection (e.g., false positives), they do not provide any recommendations or strategies for mitigating these risks.