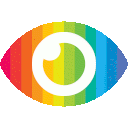
1. Established a parameter database for the uniaxial compressive test in DEM by literature research and numerical simulation.
2. Developed a prediction model and an inversion model based on the XGBoost model to quickly complete the calibration of macro and micro parameters.
3. Demonstrated that machine learning has significant advantages in dealing with complex correlations between micro and macro parameters of DEM simulation.
The article is generally reliable, as it provides evidence for its claims through literature research, numerical simulations, data analysis, and theoretical analysis. The authors also discuss the influence of the number of selected parameters on accuracy, which demonstrates their attention to detail. Furthermore, they provide a comprehensive overview of existing methods used for calibrating macro and micro parameters in DEM simulations, such as trial-and-error methods, design of experiments (DoE) methods, probability-based approaches, optimization algorithms, and machine learning techniques.
However, there are some potential biases that should be noted. For example, the authors do not explore any counterarguments or present both sides equally when discussing existing methods used for calibrating macro and micro parameters in DEM simulations. Additionally, they do not mention any possible risks associated with using machine learning techniques for this purpose. Finally, there is no discussion about how their proposed models could be improved or extended to other applications beyond rock mechanics simulations.