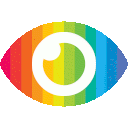
1. The study presents a computational approach using deep learning to predict cancer outcomes from digital pathology images.
2. The survival convolutional neural networks (SCNNs) integrate information from histology images and genomic biomarkers to predict time-to-event outcomes with higher accuracy than current clinical methods.
3. SCNNs recognize important structures in histology images that are related to prognosis, providing insights into the prediction mechanisms of deep learning models.
The article titled "Predicting cancer outcomes from histology and genomics using convolutional networks" discusses a computational approach for predicting patient outcomes in cancer using deep learning techniques. The authors propose the use of survival convolutional neural networks (SCNNs) that integrate information from histology images and genomic biomarkers to predict time-to-event outcomes.
One potential bias in this article is the lack of discussion on the limitations and challenges of using deep learning algorithms for predicting cancer outcomes. While the authors briefly mention challenges such as tumor heterogeneity and the need for large training cohorts, they do not provide a comprehensive analysis of these limitations. Deep learning algorithms require large amounts of labeled data to train effectively, which may not always be available in medical applications. Additionally, deep learning models are often considered "black box" models, making it difficult to interpret their prediction mechanisms. These limitations should have been discussed in more detail to provide a balanced view of the potential risks and challenges associated with using SCNNs.
Another potential bias is the lack of comparison with other existing methods for predicting cancer outcomes. The authors claim that their approach surpasses the current clinical paradigm for predicting overall survival in patients diagnosed with glioma, but they do not provide evidence or comparisons with other methods to support this claim. It would have been beneficial to include a comparative analysis with traditional survival models or other machine learning approaches to validate the superiority of SCNNs.
Furthermore, there is a lack of discussion on potential ethical considerations and implications of using deep learning algorithms in precision medicine. Deep learning algorithms rely on large amounts of patient data, including sensitive genomic information, which raises concerns about privacy and data security. The article does not address these ethical considerations or discuss any measures taken to ensure patient confidentiality.
Additionally, while the article highlights the advantages of deep learning in medical imaging tasks, it fails to acknowledge some potential drawbacks. Deep learning algorithms are computationally intensive and require significant computational resources for training and inference. This can be a limitation in resource-constrained healthcare settings where access to high-performance computing infrastructure may be limited.
Overall, the article presents an interesting approach for predicting cancer outcomes using deep learning techniques. However, it lacks a comprehensive analysis of the limitations and challenges associated with these methods, does not provide sufficient evidence to support its claims, and fails to address potential ethical considerations. A more balanced discussion of these aspects would have strengthened the article's credibility and provided a more complete picture of the potential implications of using SCNNs in clinical practice.