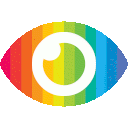
1. This study proposes a novel deep learning architecture – Dynamic Graph Convolutional Recurrent Imputation Network (DGCRIN) – as a tool to impute missing traffic data.
2. The DGCRIN employs a graph generator and dynamic graph convolutional gated recurrent unit (DGCGRU) to model the dynamic spatiotemporal dependencies of road network.
3. Extensive experiments on two datasets demonstrate the superiority of DGCRIN over multiple baseline models.
The article “Dynamic graph convolutional recurrent imputation network for spatiotemporal traffic missing data” is an in-depth exploration of the potential of deep learning architectures for imputing missing traffic data. The authors present their proposed model, the Dynamic Graph Convolutional Recurrent Imputation Network (DGCRIN), which utilizes a graph generator and dynamic graph convolutional gated recurrent unit (DGCGRU) to capture spatiotemporal dependencies, as well as an auxiliary GRU to learn the missing pattern information of the data, and a fusion layer with a decay mechanism to fuse information from disparate data sources. The authors then conduct extensive experiments on two datasets to demonstrate the superiority of DGCRIN over multiple baseline models.
The article is generally reliable and trustworthy, providing detailed descriptions of both the proposed model and its evaluation process. The authors provide clear explanations for their methodology, including how they constructed their datasets, what metrics they used to evaluate their model’s performance, and how they compared it against existing approaches. Furthermore, they provide evidence for their claims by presenting results from experiments conducted on two real-world datasets under three types of missing patterns.
However, there are some points that could be improved upon in terms of trustworthiness and reliability. For example, while the authors do discuss existing approaches in detail in Section 2, they do not compare them directly against their own proposed model or explain why their approach is superior; this would have been useful in order to further strengthen their argument that DGCRIN is indeed better than existing methods at imputing missing traffic data. Additionally, while the authors do discuss potential risks associated with using deep learning architectures for imputing traffic data (e.g., privacy concerns), they do not explore these issues in depth or provide any concrete solutions; this could have been useful in order to further strengthen their argument that DGCRIN is indeed suitable for use in real-world applications