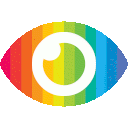
1. Online customer reviews have a significant impact on purchase decisions in the e-commerce era, and customers rely on them to gather information about potential purchases.
2. The perceived usefulness of online reviews is influenced by both central factors (such as review content) and peripheral factors (such as star rating, review length, and total number of votes).
3. The impact of these factors varies depending on the type of product, with peripheral sources having a more significant impact on search products compared to experience products.
The article titled "Perceived usefulness of online customer reviews: A review mining approach using machine learning & exploratory data analysis" discusses the impact of online customer reviews on purchase decisions and explores the factors that influence the perceived usefulness of these reviews. While the article provides valuable insights into the topic, there are several areas where it could be improved.
One potential bias in the article is its focus on positive aspects of online customer reviews and their impact on purchase decisions. The article emphasizes the importance of helpful reviews and how they can facilitate future purchases. However, it does not adequately address the potential drawbacks or limitations of relying solely on online reviews for making purchase decisions. For example, there is no discussion about fake or manipulated reviews, which can mislead consumers and undermine the credibility of online platforms.
Another limitation of the article is its narrow focus on three specific products (videogame, digital music, and grocery item) from Amazon.com. While these products may be representative of certain categories, they may not capture the full range of products available online. This limits the generalizability of the findings and raises questions about whether similar patterns would emerge for other types of products.
The article also lacks a comprehensive analysis of potential biases in online customer reviews. It briefly mentions factors such as review content, star rating, review length, and total number of votes as sources of influence on perceived usefulness. However, it does not delve into how these factors can introduce biases in reviews or affect consumers' interpretation of them. For example, high ratings or positive sentiment may lead to confirmation bias, where consumers only pay attention to information that supports their preconceived notions.
Additionally, while the article acknowledges that there are mixed results in previous research regarding determinants of perceived review helpfulness, it does not thoroughly explore these inconsistencies or provide a clear explanation for them. This leaves readers with unanswered questions about why different studies have found conflicting results and what factors might contribute to these discrepancies.
Furthermore, the article does not adequately address potential risks or limitations of using machine learning and exploratory data analysis in review mining. It does not discuss issues such as algorithmic bias, privacy concerns, or the limitations of automated sentiment analysis. These are important considerations when analyzing large volumes of user-generated content and should be acknowledged and addressed in the article.
Overall, while the article provides some valuable insights into the perceived usefulness of online customer reviews, it has several limitations and areas for improvement. A more balanced and comprehensive analysis of biases, limitations, and potential risks would enhance the credibility and relevance of the research. Additionally, expanding the scope beyond a few specific products would increase the generalizability of the findings.