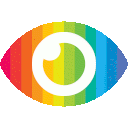
1. ChatGPT is OpenAI's language transformer model that uses self-attention to mimic human cognitive function in predicting human language.
2. Transformers have soft weights, which can be changed at runtime, and embeddings give more power and capability to these soft weights by creating a low-dimensional space that factors into the final calculation of the model.
3. Embeddings help categorize words and capture their meaning in a vector representation, allowing for more accurate language model predictions. ChatGPT uses sub-word embeddings to categorize and describe certain parts of words.
The article "Embeddings: ChatGPT's Secret Weapon" by Emma Boudreau provides an overview of embeddings and their role in language transformers like ChatGPT. The author explains that embeddings are a low-dimensional space that gives cadence to much larger high-dimensional vectors, which can be used as a classification to infer more about nuances in data. The article also discusses how OpenAI has its own embeddings endpoint, making it easy to perform natural language tasks.
Overall, the article provides a clear and concise explanation of embeddings and their role in language transformers. However, there are some potential biases and missing points of consideration that should be noted.
Firstly, the article focuses solely on the benefits of embeddings without exploring any potential risks or drawbacks. While embeddings can certainly improve the accuracy of language models, there may be concerns around privacy and bias if these models are used for sensitive applications such as hiring or lending decisions.
Secondly, the article does not provide any evidence or examples to support its claims about the accuracy of ChatGPT or other language transformers. While these models have produced impressive results in some cases, there is still debate among experts about their limitations and potential biases.
Finally, the article is somewhat promotional in nature, as it highlights OpenAI's embedding endpoint without discussing any alternatives or competing products. This could be seen as partiality towards OpenAI and may undermine the credibility of the article.
In conclusion, while "Embeddings: ChatGPT's Secret Weapon" provides a useful introduction to embeddings and their role in language transformers, readers should approach it with some caution due to its potential biases and one-sided reporting. It would be beneficial for future articles on this topic to explore both sides of the debate around language transformers and provide more evidence to support their claims.