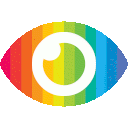
1. Machine learning algorithms were developed to predict individual occupant's behavior in manual control of windows for cross-ventilation in homes.
2. The proposed algorithms showed better predictive performance compared to logistic regression models and can help understand the influence of occupant behavior on indoor environments in buildings.
3. The driving parameters of manual control of windows in each sample home can be clearly drawn by the algorithms, which can contribute to the modeling of occupants' behavior and its impact on energy use and indoor environments.
The article "Machine learning algorithms for predicting occupants' behaviour in the manual control of windows for cross-ventilation in homes" by Junseok Park et al. presents a methodology for predicting individual occupant's behavior related to the manual control of windows using machine learning algorithms. The study aims to understand the influence of occupant behavior on indoor environments in buildings and identify driving parameters for window opening and closing behavior in each sample home.
The article provides a comprehensive review of previous studies on occupants' adaptive behaviors, including adjusting windows and blinds, thermostat set points, clothing levels, and the use of lights, fans, and HVAC systems. The authors highlight the importance of understanding occupants' behavior as it can significantly impact indoor environments and energy use in buildings.
The study uses field monitoring data from 23 sample homes located in Seoul and suburban areas. The authors propose six machine learning algorithms to predict occupant behavior related to window opening and closing. They compare the predictive performance of these algorithms with that of a logistic regression model. Among the algorithms tested, K-Nearest Neighbors (KNN) showed the best fitness with the monitored data set.
The article highlights the limitations of logistic regression models in explaining diversities and differences in driving variables for window opening and closing behavior. Machine learning algorithms are better suited to interpret stochastic and non-linear relationships between manual control of windows and variables of both indoor and outdoor environments.
While the study provides valuable insights into predicting individual occupant behavior related to window opening and closing, there are some potential biases that need consideration. The sample size is relatively small, consisting only of 23 homes located in Seoul and suburban areas. Therefore, it may not be representative of other regions or building types.
Additionally, while machine learning algorithms are useful for interpreting non-linear relationships between variables, they require large amounts of data to train effectively. It is unclear whether this methodology would be practical or cost-effective for smaller-scale projects or buildings.
Overall, the article provides a useful contribution to the field of building energy efficiency and occupant behavior. However, further research is needed to validate the proposed methodology and explore its potential limitations in different contexts.