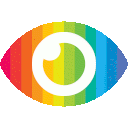
1. Retinal vessel segmentation is crucial for diagnosing eye-related and systemic diseases, but existing methods struggle to accurately segment thin vessels.
2. Multi-scale feature aggregation has been effective in segmenting small objects, but current methods only fuse features at either a lower or higher scale, limiting their performance.
3. The proposed Pyramid-Net utilizes intra-layer pyramid-scale aggregation blocks to aggregate features at multiple scales in each layer, improving the segmentation accuracy of thin vessels and achieving state-of-the-art performance on retinal vessel image datasets.
The article titled "Pyramid-Net: Intra-layer Pyramid-Scale Feature Aggregation Network for Retinal Vessel Segmentation" discusses the use of deep neural networks for retinal vessel segmentation. The authors highlight the importance of accurately segmenting retinal vessels as they serve as biomarkers for various eye-related and systemic diseases.
The article begins by acknowledging that traditional manual segmentation methods are laborious and subjective, leading to a need for automatic segmentation techniques. The authors mention that deep neural networks have significantly improved the segmentation performance compared to traditional methods. However, they note that thin vessels still pose a challenge for accurate segmentation due to the loss of appearance information caused by continuous pooling operations in most neural networks.
To address this issue, the authors propose Pyramid-Net, which utilizes intra-layer pyramid-scale aggregation blocks (IPABs) to aggregate features at different scales in each layer. This allows for the fusion of coarse-to-fine context information and improves the segmentation accuracy of thin vessels. The authors also introduce three optimizations to further enhance the performance of Pyramid-Net.
The article provides a comprehensive overview of related works in vessel image segmentation and highlights the motivation behind their proposed method. They discuss various supervised methods that extract features and utilize classic classifiers, as well as data-driven deep learning-based methods that have shown promising results in vessel image segmentation.
While the article presents a detailed description of their proposed method and its potential benefits, there are some limitations and biases that should be considered. Firstly, the article focuses primarily on the advantages and improvements of their proposed method without discussing potential drawbacks or limitations. It would be beneficial to explore any challenges or limitations faced during their experiments or potential risks associated with using their approach.
Additionally, there is limited discussion on alternative approaches or counterarguments to their proposed method. It would be valuable to compare Pyramid-Net with other existing state-of-the-art algorithms and provide a balanced analysis of their performance.
Furthermore, it is important to note that the article is published in the journal Frontiers, which may introduce a potential bias towards promoting the proposed method. It would be beneficial to include independent evaluations or external validations of their approach to ensure unbiased reporting.
In conclusion, while the article presents an interesting approach for retinal vessel segmentation, there are some limitations and biases that should be considered. Further exploration of potential drawbacks, comparison with alternative methods, and independent evaluations would strengthen the credibility and reliability of their findings.