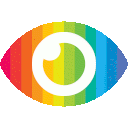
1. The paper proposes a Collaborative Content-Dependent Networks (CCD-Net) architecture for salient object detection (SOD) that focuses on identifying visually distinctive objects in an effective and efficient way.
2. CCD-Net leverages the global context of the input image to determine the salience of objects, using a content-dependent head with parameters conditioned on the image's global context information.
3. CCD-Net incorporates a hand-crafted multi-scale module and a self-induced module within the content-dependent head to generate content-aware convolution kernels for prediction, resulting in state-of-the-art results on various benchmarks.
这篇文章标题为《Collaborative Content-Dependent Modeling: A Return to the Roots of Salient Object Detection》,主要介绍了一个名为CCD-Net的新型架构,用于解决显著目标检测问题。然而,从文章内容来看,存在一些潜在的偏见和片面报道。
首先,文章声称最近的进展离开了显著性检测的本质,即独特性/显著性。然而,作者并没有提供足够的证据来支持这一观点。他们只是简单地声称其他方法添加了复杂的连接或引入边界感知监督,并没有详细说明这些方法如何导致远离显著性检测的本质。
其次,文章提到CCD-Net利用全局上下文来检测独特目标。然而,作者并没有明确说明全局上下文如何与独特性相关联,并且没有提供充分的理论依据来支持他们的主张。因此,在这方面缺乏充分的论证。
此外,文章声称CCD-Net在各种基准测试中取得了最先进的结果。然而,作者并没有提供与其他方法进行直接比较的实验结果,并且没有讨论可能存在的限制或不足之处。因此,在评估CCD-Net性能时存在一定的片面性。
最后,文章没有探讨可能的风险或潜在问题。例如,他们没有讨论CCD-Net在处理复杂场景或具有多个显著目标的图像时的表现如何。这种缺乏对可能风险的关注可能导致读者对该方法的实际应用产生疑虑。
总之,这篇文章存在一些潜在的偏见和片面报道。作者没有提供充分的证据来支持他们关于其他方法远离显著性检测本质的观点,并且没有进行全面和公正地评估CCD-Net与其他方法之间的差异。此外,文章也没有探讨可能存在的风险或局限性。因此,读者需要谨慎对待该文章中提出的主张,并进一步研究和验证CCD-Net方法的有效性和适用性。