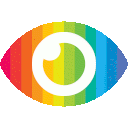
1. Sarcasm detection in Indian indigenous language Hindi is a challenge due to altered vocabulary and free-word order.
2. The Amalgam Neural Architecture (TANA) combines LSTM and SVM with word-emoji embeddings to detect sarcasm in Hindi tweets.
3. TANA achieves a F-score of 0.9675, outperforming previous works and improving sentiment analysis in Hindi.
The article titled "TANA: The amalgam neural architecture for sarcasm detection in Indian indigenous language combining LSTM and SVM with word-emoji embeddings" discusses the development of a model to detect sarcasm in Hindi tweets. The article highlights the challenges of sentiment analysis, particularly in detecting sarcasm, due to altered vocabulary and playful language mannerisms used on online forums. The authors propose a model that combines LSTM and SVM with word-emoji embeddings to detect sarcasm in Hindi tweets.
The article provides a comprehensive overview of the challenges faced in sentiment analysis and the need for more efficient models for sarcasm detection in resource-poor indigenous languages like Hindi. However, there are some potential biases and limitations to consider.
Firstly, the article focuses solely on the use of deep learning approaches for sarcasm detection, ignoring other methods such as rule-based or lexicon-based techniques. While deep learning approaches have shown promising results, it is important to consider other methods that may be more suitable for certain contexts or languages.
Secondly, the article only considers one dataset (Sarc-H) for evaluating the performance of their proposed model. While Sarc-H is a manually annotated dataset specifically designed for sarcasm detection in Hindi tweets, it may not be representative of all types of sarcastic language used on social media platforms. Therefore, it would be beneficial to evaluate the proposed model on multiple datasets to ensure its generalizability.
Thirdly, while the authors acknowledge that Hindi has free-word order, they do not explore how this affects their proposed model's performance. It would be interesting to see how their model performs when presented with different word orders or sentence structures commonly used in Hindi.
Finally, while the article acknowledges that emojis can convey emotions difficult to express through text alone and are almost universally understood by diverse audiences, it does not explore potential biases or cultural differences in emoji interpretation across different regions or communities.
In conclusion, while the article provides valuable insights into developing efficient models for sarcasm detection in resource-poor indigenous languages like Hindi using deep learning approaches combined with word-emoji embeddings, there are potential biases and limitations that need consideration. Further research is needed to explore alternative methods for sarcasm detection and evaluate proposed models on multiple datasets representing diverse types of sarcastic language used on social media platforms.