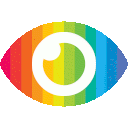
1. The article proposes a novel visual detection method, AC-SNGAN, for surface damage detection on conveyor belts using an improved ACGAN and multiple data augmentation techniques.
2. The method combines a generative adversarial network (GAN) based data augmentation module to enhance the feature database of conveyor belt surface damage samples.
3. By applying Wasserstein distance and spectral normalization strategies, the deployment time of the detection method is reduced, and it achieves high efficiency and stability in generating high-quality samples of various types of conveyor belt surface defects.
The article titled "AC-SNGAN: Multi-class Data Augmentation for Conveyor Belt Surface Damage Detection using Improved ACGAN" discusses a new visual detection method for identifying surface damage on conveyor belts. The authors propose the use of an auxiliary classifier spectral normalization generative adversarial network (AC-SNGAN) to enhance the feature database of damaged samples. They claim that this method reduces training and deployment time and achieves high-quality results.
One potential bias in this article is the lack of discussion about alternative methods for conveyor belt surface damage detection. The authors only briefly mention other techniques such as visual inspection but do not provide a comprehensive analysis or comparison with their proposed method. This omission limits the reader's understanding of the strengths and weaknesses of different approaches.
Additionally, the article lacks evidence to support some of its claims. For example, it states that the proposed method can efficiently and stably generate high-quality samples of various types of conveyor belt surface defects. However, there is no detailed explanation or empirical evidence provided to support this assertion. Without proper validation or comparison with existing methods, it is difficult to assess the effectiveness and reliability of the proposed approach.
Furthermore, the article does not explore potential counterarguments or limitations of the AC-SNGAN method. It fails to address possible challenges or drawbacks that may arise when applying this technique in real-world scenarios. This oversight undermines the credibility and completeness of the research presented.
Another concern is that the article appears to have promotional content rather than providing an objective analysis. The language used throughout the article seems biased towards promoting the proposed method as a superior solution without acknowledging any potential shortcomings or limitations.
In terms of risks, while the authors mention that timely detection and handling of conveyor belt damage are crucial to prevent further deterioration and ensure safe operation, they do not discuss any specific risks associated with false positives or false negatives in their detection system. This omission leaves out important considerations related to reliability and safety.
Overall, the article suffers from several biases and shortcomings, including one-sided reporting, unsupported claims, missing evidence, unexplored counterarguments, promotional content, and a lack of comprehensive analysis. It would benefit from a more balanced and critical examination of the proposed method and its implications in real-world applications.