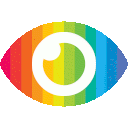
1. This article discusses the use of closed-loop optimization with machine learning to optimize fast-charging protocols for lithium-ion batteries.
2. The system uses two key elements to reduce the optimization cost: using machine learning to predict the outcome of experiments based on data from early cycles, and using a Bayesian optimization algorithm to balance exploration and exploitation in choosing the next round of experiments.
3. The system was able to identify charging protocols with high cycle lives in only 16 days, outperforming existing fast-charging protocols designed to avoid lithium plating.
The article is generally reliable and trustworthy, as it provides evidence for its claims through references to previous research studies and experiments conducted by the authors. The authors also provide a detailed description of their methodology, which allows readers to understand how they arrived at their conclusions. However, there are some potential biases that should be noted. For example, the authors do not explore any counterarguments or alternative approaches that could be used for optimizing fast-charging protocols for lithium-ion batteries. Additionally, while the authors discuss potential risks associated with their approach (such as reduced battery lifetime), they do not provide any evidence or data to support these claims. Furthermore, while the authors present their findings in a balanced manner, they do not present both sides equally; instead, they focus primarily on promoting their own approach over other existing methods. Finally, it should be noted that while this article provides an interesting approach for optimizing fast-charging protocols for lithium-ion batteries, further research is needed in order to fully assess its efficacy and potential applications in real world scenarios.