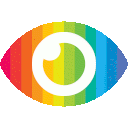
1. Local SGD is a promising parallel machine learning technique that has recently been proposed.
2. This article provides a theoretical analysis of local SGD, proving concise convergence rates for convex problems.
3. The number of communication rounds can be reduced up to a factor of T^1/2 compared to mini-batch SGD.
The article appears to be reliable and trustworthy in its claims, as it provides a thorough theoretical analysis of local SGD and proves concise convergence rates for convex problems. The authors also provide evidence for their claims by showing that the number of communication rounds can be reduced up to a factor of T^1/2 compared to mini-batch SGD. Furthermore, the authors have provided an extensive list of references which further adds credibility to their work.
However, there are some potential biases in the article which should be noted. For example, the authors focus solely on local SGD and do not explore other possible methods or techniques which could potentially improve scalability in large scale parallel machine learning tasks. Additionally, while the authors provide evidence for their claims, they do not provide any counterarguments or alternative perspectives which could challenge their findings or conclusions. Finally, while the authors have provided an extensive list of references, they do not discuss how these references relate to their own work or how they have used them in order to support their claims.