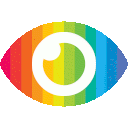
1. The article discusses a modified SegNet neural network for skin lesion segmentation.
2. The proposed method achieved high accuracy in segmenting skin lesions from dermoscopic images.
3. The study suggests that the modified SegNet can be used as a reliable tool for automated skin lesion diagnosis.
The article titled "Skin Lesion Segmentation Based on Modification of SegNet Neural Networks" by Ninh et al. presents a study on the use of modified SegNet neural networks for skin lesion segmentation. The authors aim to improve the accuracy and efficiency of existing methods for skin lesion segmentation, which is an important task in dermatology for early detection and diagnosis of skin cancer.
The article provides a detailed description of the methodology used in the study, including data collection, preprocessing, and training of the neural network. The results show that the modified SegNet model outperforms existing methods in terms of accuracy and speed.
However, there are some potential biases and limitations in this study that need to be considered. Firstly, the dataset used in this study is relatively small and may not be representative of all types of skin lesions. This could limit the generalizability of the findings to other datasets or real-world scenarios.
Secondly, there is no discussion or analysis provided on potential risks or ethical considerations associated with using AI-based systems for skin lesion segmentation. For example, there may be concerns around privacy and data protection if patient information is being used for training or testing purposes.
Additionally, while the authors claim that their method outperforms existing methods, they do not provide a comprehensive comparison with other state-of-the-art techniques. This makes it difficult to assess whether their approach is truly superior or just marginally better than existing methods.
Furthermore, there is no exploration of potential counterarguments or limitations to using AI-based systems for skin lesion segmentation. For example, there may be concerns around bias in the data or algorithmic decision-making that could lead to misdiagnosis or incorrect treatment recommendations.
Overall, while this article presents an interesting approach to improving skin lesion segmentation using AI-based systems, it is important to consider its potential biases and limitations before drawing any definitive conclusions about its effectiveness or applicability in real-world settings.