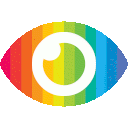
1. A study aimed to differentiate between chronic obstructive pulmonary disease (COPD) and asthma using quantitative CT imaging and machine learning.
2. The study found that a subset of seven CT features could differentiate between COPD and asthma with moderate-to-high accuracy.
3. This research can help identify potential "treatable traits" in patients with overlapping disease features, allowing for more personalized treatment strategies.
The article titled "Differentiating COPD and asthma using quantitative CT imaging and machine learning" presents a study that aims to determine the optimal subset of CT imaging features for differentiating between chronic obstructive pulmonary disease (COPD) and asthma using machine learning. While the study provides valuable insights into the potential use of CT imaging and machine learning in distinguishing between these two diseases, there are several aspects of the article that warrant critical analysis.
One potential bias in the article is the selection of participants from a single hospital, Heidelberg University Hospital in Germany. This may limit the generalizability of the findings to other populations or healthcare settings. Additionally, the sample size of 95 participants (48 with COPD and 47 with asthma) is relatively small, which could affect the statistical power and reliability of the results.
The article claims that COPD and asthma can be differentiated using machine learning with moderate-to-high accuracy based on a subset of seven CT features. However, it does not provide detailed information about how these features were selected or how they contribute to the differentiation process. Without this information, it is difficult to assess the validity and robustness of the findings.
Furthermore, while the article acknowledges that some patients may have features of both COPD and asthma (asthma-COPD overlap), it does not explore how well machine learning can differentiate these overlapping cases. This is an important consideration given that distinguishing between these conditions can be challenging in clinical practice.
The article also mentions that emphysema is a treatable trait associated with accelerated lung function decline in patients with asthma. However, it does not provide evidence or references to support this claim. Without supporting evidence, readers may question the validity of this statement.
Another limitation of the study is its focus on CT imaging features alone without considering other clinical factors or biomarkers that could aid in differentiating COPD and asthma. By solely relying on CT imaging, important information from other diagnostic tests or patient characteristics may be overlooked.
Additionally, the article does not discuss potential risks or limitations associated with using machine learning in this context. For example, there may be concerns about the interpretability and generalizability of machine learning models, as well as the potential for bias in the training data.
Overall, while the study presented in the article offers insights into the use of CT imaging and machine learning for differentiating COPD and asthma, there are several limitations and missing points of consideration that should be addressed. Further research with larger sample sizes and a more comprehensive approach considering multiple diagnostic factors is needed to validate these findings and provide a more complete understanding of how to differentiate between these two diseases.