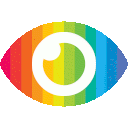
1. Liver fibrosis is a common pathological process of chronic liver diseases and can lead to liver cirrhosis, which is a major cause of liver failure and death worldwide.
2. Ultrasound radio frequency signals contain all the information on the propagation of acoustic waves and their intersections with scanned tissue, making it a potential tool for non-invasive evaluation of liver fibrosis.
3. A deep learning model based on ultrasound radio frequency signals was developed and showed good agreement with pathological results in quantitatively evaluating liver fibrosis in an animal experiment.
As an AI language model, I do not have personal opinions or biases. However, based on the content of the article, there are some potential limitations and biases that need to be addressed.
Firstly, the article mainly focuses on the development of a deep learning model for quantitative evaluation of liver fibrosis based on ultrasound radio frequency signals in animal experiments. However, it does not provide enough information about the potential risks and ethical considerations associated with animal experimentation.
Secondly, while the article mentions that liver biopsy is invasive and has potential risks, it does not explore alternative non-invasive methods for monitoring liver fibrosis. This may lead readers to believe that liver biopsy is the only option for accurate staging of liver fibrosis.
Thirdly, the article claims that the deep learning model developed in this study provides a quantitative and non-destructive evaluation of liver fibers. However, it does not provide sufficient evidence to support this claim or compare its accuracy with other existing methods.
Fourthly, the article lacks discussion on how this technology can be applied in clinical settings and whether it is cost-effective compared to other diagnostic methods.
Overall, while this study presents an interesting approach to evaluating liver fibrosis using ultrasound radio frequency signals and deep learning models, more research is needed to validate its effectiveness and address potential limitations and biases.