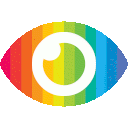
1. The paper investigates the use of deep convolutional neural networks (CNNs) for airport detection in remote sensing images (RSIs).
2. CNNs are found to be effective in airport detection, as they ensure a tuned feature vector and yield better classification accuracy.
3. The proposed method utilizes a CNN architecture with five convolutional layers and three fully connected layers, achieving an accuracy of 95.21% in airport detection.
The article titled "Deep Convolutional Neural Networks for Airport Detection in Remote Sensing Images" discusses the usage of deep convolutional neural networks (CNNs) for airport detection in remote sensing images. The authors claim that CNNs have gained attention in recent years and have been successfully applied in various computer vision applications. They argue that CNNs not only ensure a tuned feature vector but also yield better classification accuracy compared to traditional methods.
The article starts by providing an overview of the problem of airport detection in remote sensing images and the existing approaches, which are mainly based on pattern recognition and feature extraction. The authors then introduce their proposed method, which involves using CNNs to detect candidate regions on remote sensing images and train a CNN architecture for airport detection. They describe the architecture of their CNN model, including convolutional layers, fully connected layers, normalization layers, dropout layers, and data augmentation strategy.
The authors claim that their proposed method outperforms previously proposed methods with an accuracy of 95.21%. However, they do not provide any evidence or detailed analysis to support this claim. They mention that several experiments were performed to evaluate the performance of the CNN but do not provide any specific details about these experiments or their results.
One potential bias in this article is the lack of discussion about potential limitations or challenges of using CNNs for airport detection in remote sensing images. While the authors mention that data augmentation was used to reduce overfitting, they do not discuss other potential issues such as dataset bias, generalization to different types of airports or environmental conditions, or computational requirements.
Another limitation is the lack of comparison with other state-of-the-art methods or benchmarks. The authors only mention that comparative work validated the efficiency of their proposed method without providing any details about this comparison or references to previous work.
Additionally, there is a lack of discussion about potential ethical considerations or risks associated with using deep learning algorithms for airport detection. For example, there could be concerns about privacy or security implications if these algorithms are used for surveillance purposes.
Overall, while the article presents an interesting application of CNNs for airport detection in remote sensing images, it lacks detailed analysis, evidence, and discussion of potential limitations or ethical considerations. Further research and experimentation are needed to validate the claims made in this article and address the missing points of consideration.