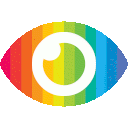
1. Researchers used unsupervised learning to train a deep contextual language model on 86 billion amino acids across 250 million protein sequences, resulting in a model that contains information about biological properties in its representations.
2. The learned representation space has a multiscale organization reflecting structure from the level of biochemical properties of amino acids to remote homology of proteins, and information about secondary and tertiary structure is encoded in the representations.
3. Representation learning produces features that generalize across a range of applications, enabling state-of-the-art supervised prediction of mutational effect and secondary structure and improving state-of-the-art features for long-range contact prediction.
The article "Biological structure and function emerge from scaling unsupervised learning to 250 million protein sequences" presents a study on the use of unsupervised learning to train a deep contextual language model on 86 billion amino acids across 250 million protein sequences. The authors claim that the resulting model contains information about biological properties in its representations, and that representation learning produces features that generalize across a range of applications, enabling state-of-the-art supervised prediction of mutational effect and secondary structure.
The article provides detailed information about the methodology used in the study, including the data sources, the architecture of the deep contextual language model, and the evaluation metrics used to assess its performance. The authors also present several visualizations of the learned representations, which they argue demonstrate their ability to capture biochemical properties of amino acids and structural features of proteins.
While the article provides a thorough description of the study's methods and results, it is important to note that it is written from a highly technical perspective and may be difficult for non-experts to understand. Additionally, there are several potential biases and limitations to consider when interpreting the findings.
One potential bias is that the study was conducted by researchers affiliated with Microsoft Research, which may have influenced their approach or interpretation of results. Additionally, while the authors claim that their method produces representations that generalize well across different applications, it is unclear how well these representations would perform on datasets outside of those used in this study.
Another limitation is that while the authors provide evidence for their claims about the ability of their method to capture biochemical properties and structural features of proteins, they do not explore potential counterarguments or alternative explanations for these findings. For example, it is possible that some aspects of protein structure or function are not captured by sequence data alone.
Overall, while this article presents an interesting study on using unsupervised learning to learn representations of protein sequences, readers should be aware of potential biases and limitations when interpreting its findings.