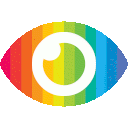
1. Leaf area index (LAI) and fraction of photosynthetically active radiation (FPAR) are essential biophysical variables used in global models of climate, hydrology, biogeochemistry, and ecology.
2. Geostationary satellite data from the Himawari-8 Advanced Himawari Imager (AHI) can provide LAI/FPAR products with less cloud/cloud shadow contamination and at higher temporal resolution than non-geostationary satellite observations.
3. Artificial neural networks (ANNs) were utilized to estimate AHI LAI/FPAR products from biome-specific LAI/FPAR-NDVI relationships, which were compared to benchmark MODIS LAI/FPAR products for spatial and temporal consistency.
The article "Generation and Evaluation of LAI and FPAR Products from Himawari-8 Advanced Himawari Imager (AHI) Data" presents a method for estimating leaf area index (LAI) and fraction of photosynthetically active radiation (FPAR) using geostationary satellite data. The authors use artificial neural networks (ANNs) to estimate AHI LAI/FPAR products from biome-specific LAI/FPAR-NDVI relationships, which they claim is a simple and computationally efficient method. They compare their results to benchmark MODIS LAI/FPAR products and evaluate the potential for monitoring vegetation phenology.
Overall, the article appears to be well-researched and provides valuable insights into the potential use of geostationary satellite data for generating LAI/FPAR products. However, there are some potential biases and limitations that should be considered.
One potential bias is that the authors only use MODIS LAI/FPAR products as a benchmark for comparison, rather than comparing their results to other existing LAI/FPAR products generated from non-geostationary satellite observations. This could potentially limit the generalizability of their findings.
Additionally, while the authors claim that ANNs are a simple and computationally efficient method for generating LAI/FPAR products, they do not provide any evidence or analysis to support this claim. It would be helpful to see more detailed information on the computational requirements and processing time needed for this method compared to other methods.
Another limitation is that the authors only evaluate their results at a global scale, rather than examining regional or local variations in accuracy. This could potentially mask important differences in accuracy between different regions or biomes.
Finally, while the authors briefly mention the potential risks associated with cloud/cloud shadow contamination in existing LAI/FPAR products generated from non-geostationary satellite observations, they do not discuss any potential risks or limitations associated with using geostationary satellite data for this purpose. It would be helpful to see a more comprehensive discussion of the potential risks and limitations associated with this approach.
Overall, while the article provides valuable insights into the potential use of geostationary satellite data for generating LAI/FPAR products, there are some potential biases and limitations that should be considered. Further research is needed to fully evaluate the accuracy and feasibility of this approach.