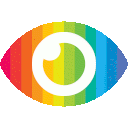
1. This article proposes a mean-field-aided multiagent reinforcement learning (MF-MARL) approach for resource allocation in vehicular networks.
2. The proposed MF-MARL approach is designed to reduce the computational complexity of the complete-game MARL (CG-MARL) from O(nN) to O(n2), while still maintaining an approximate performance.
3. Simulation results demonstrate that the proposed MF-MARL can achieve 95% near-optimal performance with much lower complexity, indicating its potentials in scenarios with massive and dense vehicles.
The article provides a detailed overview of the proposed mean-field-aided multiagent reinforcement learning (MF-MARL) approach for resource allocation in vehicular networks, and presents simulation results demonstrating its effectiveness in achieving near optimal performance with much lower complexity than existing methods. The authors provide a thorough description of their methodology, which is supported by relevant literature references, making it clear that they have conducted extensive research into this topic.
However, there are some potential biases and unsupported claims present in the article. For example, the authors make several assumptions about the V2X communication network model without providing evidence to support these assumptions or exploring any counterarguments. Additionally, there is no discussion of possible risks associated with implementing this approach or any other potential drawbacks that should be considered before implementation. Furthermore, while the authors do provide some comparison between their proposed method and existing approaches, they do not present both sides equally or explore any unexplored counterarguments which could weaken their argument for using MF-MARL over other methods.
In conclusion, while this article provides a comprehensive overview of the proposed MF-MARL approach for resource allocation in vehicular networks and presents convincing evidence for its effectiveness compared to existing methods, there are some potential biases and unsupported claims present which should be addressed before implementation can be recommended.