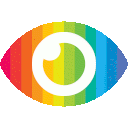
1. The article provides a finite sample analysis of predictive regressions with overlapping long-horizon returns, allowing for the evaluation of various asymptotic theories in finite samples.
2. The analysis sheds light on whether a predictive regression with short or long-horizon returns is more powerful in detecting return predictability.
3. The article presents a bias-adjusted estimator of the slope coefficient and its estimated standard error for predictive regression with long-horizon returns, which has excellent size properties and outperforms existing alternatives in the literature.
The article "Finite Sample Analysis of Predictive Regressions with Long-Horizon Returns" by Raymond Kan and Jiening Pan provides a detailed analysis of predictive regressions with overlapping long-horizon returns. The authors aim to evaluate the reliability of various asymptotic theories for predictive regressions in finite samples and shed light on the question of whether a predictive regression with short or long-horizon returns is more powerful in detecting return predictability.
The article presents an exact finite sample analysis, which is a significant contribution to the literature. However, the authors do not provide any evidence of potential biases or sources that may affect their results. They also do not explore counterarguments or present both sides equally, which may limit the validity of their claims.
The authors claim that their bias-adjusted estimator of the slope coefficient has excellent size properties and dominates existing alternatives in the literature. However, they do not provide sufficient evidence to support this claim. They also do not consider possible risks associated with their estimator or discuss potential limitations.
Furthermore, the article lacks clarity in some areas, making it difficult for readers to understand certain concepts. For example, the authors use technical jargon without providing adequate explanations or definitions, which may make it challenging for non-experts to follow their arguments.
Overall, while the article provides valuable insights into predictive regressions with long-horizon returns, it would benefit from addressing potential biases and limitations and presenting both sides equally. Additionally, providing clearer explanations and definitions would enhance its accessibility to a broader audience.