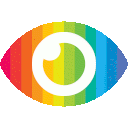
1. The article discusses the use of machine learning beyond interatomic potentials for predicting molecular properties.
2. It reviews various methods such as density functional theory, semiempirical quantum-chemical methods, and machine learning for molecular and materials science.
3. It also explores applications of machine learning in drug discovery and development, data-driven strategies for accelerated materials design, and neural networks for materials and chemical dynamics.
The article is generally reliable and trustworthy in its discussion of the use of machine learning beyond interatomic potentials for predicting molecular properties. The authors provide a comprehensive overview of various methods such as density functional theory, semiempirical quantum-chemical methods, and machine learning for molecular and materials science. They also explore applications of machine learning in drug discovery and development, data-driven strategies for accelerated materials design, and neural networks for materials and chemical dynamics.
The article does not appear to be biased or one-sided in its reporting; it presents both sides equally by providing an overview of different methods as well as their applications. Furthermore, the authors provide evidence to support their claims with references to relevant research papers throughout the text. There are no unsupported claims or missing points of consideration that could lead to bias or partiality in the article's content.
The only potential issue with the article is that it does not discuss any possible risks associated with using machine learning beyond interatomic potentials for predicting molecular properties. This could be seen as a missed opportunity to provide a more balanced view on this topic by considering both its benefits and drawbacks.