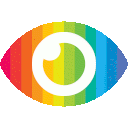
1. The paper presents a comparison of machine learning algorithms for skin cancer diagnosis based on image analysis.
2. Two approaches were used: one based on feature extraction and classification using classical machine learning algorithms, and the other using deep learning algorithms (CNNs) that perform both feature extraction and classification in one algorithm.
3. The study analyzed six classical machine learning algorithms and four CNNs, evaluated their effectiveness using common metrics, and compared their computational complexity in the context of implementation on mobile devices with limited computing performance.
The article "Comparison of Machine Learning Algorithms Used for Skin Cancer Diagnosis" presents a comparison of machine learning algorithms used for skin cancer diagnosis based on analyses of skin lesion photos. The article provides two approaches to solving the problem of automatic melanoma diagnosis: one based on the extraction of features from images using simple feature descriptors and then using selected machine learning algorithms for classification, and the other approach uses selected algorithms belonging to the subgroup of machine learning—deep learning, i.e., convolutional neural networks (CNN), which perform both feature extraction and classification in one algorithm.
The article provides a detailed description of the materials used in the research, including image data from the ISIC database, which includes data from two categories—benign and malignant skin lesions. The authors also describe their proposed research method, which involves preprocessing and extracting features vectors from input images, preparing combinations of features vectors obtained by means of individual descriptors, and carrying out the image classification process.
While the article provides a comprehensive analysis of different machine learning algorithms used for skin cancer diagnosis, it has some potential biases. For example, the article focuses mainly on classical (not-deep) machine learning algorithms and only briefly mentions deep neural networks. This bias may be due to limitations such as worse performance and scalability associated with deep neural networks. However, this bias may limit readers' understanding of current trends in machine learning for skin cancer diagnosis.
Additionally, while the authors provide a detailed description of their proposed research method, they do not discuss potential limitations or drawbacks associated with their approach. For example, they do not mention any potential risks associated with relying solely on image data for skin cancer diagnosis or any limitations associated with using specific feature descriptors.
Overall, while the article provides valuable insights into different machine learning algorithms used for skin cancer diagnosis based on analyses of skin lesion photos, readers should be aware of potential biases and limitations associated with the study's methodology.