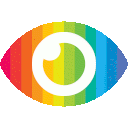
1. Reinforcement learning (RL) is a method for teaching artificial agents to make optimal decisions by interacting with an environment and receiving rewards. However, RL struggles in partially observable environments where not all factors are observable or well understood.
2. Reward machines (RMs) provide a structured representation of a reward function and can serve as memory for RL agents in partially observable environments. Previous methods required handcrafted RMs, but this work proposes a method for learning RMs directly from experience.
3. The authors propose a discrete optimization problem for learning RMs in partially observable environments and compare different methodologies to solve this problem. They also show that integrating the learned RMs into the agent-environment interaction loop significantly improves performance compared to deep RL baselines using recurrent neural networks as memory.
对于这篇文章的批判性分析,我们可以注意到以下几个方面:
1. 偏见及其来源:文章中存在一些偏见,主要体现在对强化学习方法的过度赞美和对其他方法的贬低上。作者将深度强化学习方法描述为解决复杂环境问题的最佳选择,并声称其他方法在部分可观察环境下表现不佳。然而,作者没有提供足够的证据来支持这些主张,并且忽略了其他可能有效的方法。
2. 片面报道:文章只关注了使用奖励机制作为记忆工具来解决部分可观察环境中的强化学习问题。然而,这种方法并不适用于所有情况,并且可能存在其他更好的解决方案。文章没有提供对比研究或讨论其他可能的方法。
3. 缺失的考虑点:文章没有充分考虑奖励机制在实际应用中可能面临的挑战和限制。例如,如何设计一个合适的奖励函数以达到预期目标,如何处理奖励信号稀疏或噪声等问题。
4. 所提出主张的缺失证据:尽管作者声称使用奖励机制可以显著提高性能,但文章没有提供充分的实验证据来支持这一主张。缺乏实验结果和对比研究使得读者难以评估该方法的有效性。
5. 未探索的反驳:文章没有探讨可能存在的反驳观点或批评意见。这种单方面的陈述可能导致读者对问题的全面理解和评估。
6. 宣传内容:文章中存在一些宣传性语言,试图将奖励机制描述为解决部分可观察环境中强化学习问题的最佳选择。这种宣传性语言可能会误导读者,并使他们对其他方法产生误解或忽视。
综上所述,这篇文章存在一些潜在偏见、片面报道、无根据的主张和缺失考虑点等问题。读者应该保持批判思维,并在阅读时注意到这些问题。