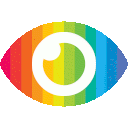
1. Diffusion models are powerful generative models used in text-conditioned image generation.
2. Variational Diffusion Models (VDM) can be derived as a special case of a Markovian Hierarchical Variational Autoencoder, with three key assumptions enabling tractable computation and scalable optimization of the ELBO.
3. Optimizing a VDM involves learning a neural network to predict one of three potential objectives: the original source input from any arbitrary noisification of it, the original source noise from any arbitrarily noisified input, or the score function of a noisified input at any arbitrary noise level.
本文是一篇关于扩散模型的综述性文章,旨在对这种生成模型进行解释、统一和深入理解。文章首先介绍了扩散模型的基本概念和应用,然后从变分和得分两个角度出发,对其进行了详细的分析和比较。
然而,在阅读本文时,我们也可以发现一些潜在的偏见和不足之处。首先,文章似乎过于强调了扩散模型的优点和应用,而忽略了其局限性和风险。例如,在介绍扩散模型作为生成模型时,文章没有提到其可能存在的过拟合问题或样本外泛化能力不足等问题。
此外,在讨论扩散模型的优化方法时,文章似乎过于简化了问题,并没有考虑到实际应用中可能遇到的复杂情况。例如,在实际应用中,如何选择合适的噪声分布、如何处理长序列数据等问题都需要进一步探讨。
最后,尽管文章试图从多个角度对扩散模型进行全面解释和比较,但仍然存在一些片面报道或缺失考虑点的情况。例如,在讨论得分方法时,文章只提到了 Tweedie's Formula,而没有涉及其他可能的得分方法或其优缺点。
综上所述,虽然本文对扩散模型进行了详细的解释和比较,但仍然存在一些潜在的偏见和不足之处。因此,在阅读本文时,我们需要保持批判性思维,并结合实际应用情况进行深入探讨。