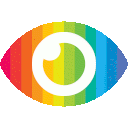
1. Emotional responses are important in human-computer conversational systems, and using emotional information improves user satisfaction and makes responses more human-like.
2. The proposed model, DC-DEDEN, introduces a dynamic emotion dictionary module to generate emotional expressions and a dual-copying mechanism to take advantage of both the emotion dictionary and words appearing in the input text.
3. Experimental results show that DC-DEDEN outperforms other state-of-the-art models in several metrics, including BLEU, embedding similarity, Rouge-L, and quality of human evaluation.
The article titled "Dual-copying mechanism and dynamic emotion dictionary for generating emotional responses" discusses the importance of generating emotional responses in human-computer conversational systems. The authors propose a model that utilizes a dynamic emotion dictionary module and a dual-copying mechanism to generate emotional expressions that are related to the dataset and sensitive to semantic importance.
The article provides a comprehensive overview of previous studies on emotional conversational systems, including early research that used manually prepared rules to choose responses with proper emotions from a dialog corpus. The authors also discuss recent studies that have utilized recurrent neural networks (RNNs) and its variants to construct conversational systems, such as chatbots and intelligent customer service.
However, the article has some potential biases and missing points of consideration. For example, the authors do not discuss the potential risks associated with emotional conversational systems, such as the possibility of reinforcing harmful stereotypes or biases. Additionally, while the authors mention previous studies that have explored explicit ways to generate emotional responses using an external emotion dictionary, they do not provide enough evidence to support their claim that using fixed and unrelated emotion dictionaries limits the overall performance of recent models.
Furthermore, while the authors propose a coherent structure to address existing problems in emotional response generation, they do not explore counterarguments or alternative approaches. For instance, they do not consider whether it might be more effective to use machine learning algorithms to learn emotional expressions directly from data rather than relying on pre-defined emotion dictionaries.
Overall, while the article provides valuable insights into emotional response generation in conversational systems, it could benefit from more balanced reporting and exploration of alternative approaches.