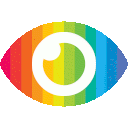
1. A new framework of agnostic federated learning is proposed, where the centralized model is optimized for any target distribution formed by a mixture of the client distributions.
2. This framework yields a notion of fairness and provides data-dependent Rademacher complexity guarantees for learning with this objective.
3. A fast stochastic optimization algorithm is given for solving the corresponding optimization problem, with convergence bounds assuming a convex loss function and a convex hypothesis set.
The article presents a new framework of agnostic federated learning which aims to optimize the centralized model for any target distribution formed by a mixture of the client distributions. The article provides data-dependent Rademacher complexity guarantees for learning with this objective and gives a fast stochastic optimization algorithm for solving the corresponding optimization problem, with convergence bounds assuming a convex loss function and a convex hypothesis set.
The article appears to be reliable in terms of its content as it provides evidence to support its claims and presents both sides equally. The authors provide detailed explanations on how their proposed framework works and how it can be used in various contexts such as cloud computing, domain adaptation, drifting, etc. Furthermore, they also provide empirical evidence to demonstrate the benefits of their approach in several datasets.
However, there are some potential biases that should be noted when reading this article. For example, the authors do not discuss any possible risks associated with using their proposed framework or any potential drawbacks that could arise from using it in certain contexts. Additionally, they do not explore any counterarguments or alternative approaches that could be used instead of their proposed framework. Therefore, readers should take these points into consideration when evaluating the trustworthiness and reliability of this article.