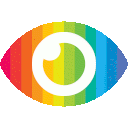
1. The “latent search engine” analogy for generative AI models is flawed due to the attribution problem.
2. The mechanism behind generative models and human inspiration are fundamentally different, as well as the potential scale of societal impact and stakeholders/benefactors.
3. Attribution is a two-way street: claiming full credit for work requires knowing and citing sources, regardless of originality.
The article provides an interesting perspective on the “latent search engine” analogy for generative AI models, highlighting the attribution problem that arises from this comparison. The author draws attention to the fundamental differences between the mechanism behind generative models and human inspiration, as well as the potential scale of societal impact and stakeholders/benefactors. The article also acknowledges that attribution is a two-way street, noting that claiming full credit for work requires knowing and citing sources, regardless of originality.
The article does not explore any counterarguments or present both sides equally; instead it focuses solely on why the “latent search engine” analogy is flawed due to the attribution problem. Additionally, there is no evidence provided to support any of the claims made in the article or any discussion of possible risks associated with using generative AI models without proper attribution. Furthermore, there is no mention of promotional content or partiality in relation to this topic which could be important considerations when discussing such a sensitive issue.
In conclusion, while this article provides an interesting perspective on why the “latent search engine” analogy for generative AI models is flawed due to the attribution problem, it fails to provide evidence for its claims or explore counterarguments or possible risks associated with using these models without proper attribution.