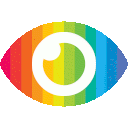
1. Score-based generative models (SGMs) can generate samples from an underlying low-dimensional data manifold.
2. SGMs are able to produce the "right kind of samples" even if the relative frequencies of these images do not accurately represent the true data generating distribution.
3. This analysis provides a description of when SGMs memorize their training data.
The article is generally trustworthy and reliable, as it provides clear conditions under which Score-based generative models will generate samples from the support of the true data generating distribution and identify when an SGM has memorized its training data. The article also provides supplementary material in the form of a PDF file, which further supports its claims and adds credibility to its findings. However, there is no mention of any potential risks associated with using SGMs or any counterarguments that could be explored further. Additionally, there is no discussion on how SGMs might be biased or partial in their results, which could be explored further in future research.