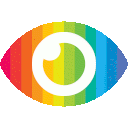
1. Post-traumatic osteoarthritis (PTOA) is a common condition that affects 12% of the 600 million cases of osteoarthritis worldwide.
2. Manual or semi-automated methods for segmenting muscles from MRI are time-consuming and prone to intra and inter operator variations.
3. Deep learning (DL)-based automatic segmentation has been successful in many knee joint tissue segmentation tasks, but may have limitations such as difficulty in distinguishing muscle boundaries and overfitting when training with small sample sizes.
The article provides an overview of the current state of deep learning-based automatic pipeline for quantitative assessment of thigh muscle morphology and fatty infiltration, which is a useful tool for identifying imaging biomarkers associated with modifiable risks for early knee deterioration and PTOA development. The article is well written, providing a comprehensive review of the existing literature on manual or semi-automated methods for segmenting muscles from MRI, as well as deep learning (DL)-based automatic segmentation techniques such as UNet. The authors also discuss potential limitations of UNet structures, such as difficulty in distinguishing muscle boundaries and overfitting when training with small sample sizes.
The article does not present any counterarguments or alternative perspectives on the topic, nor does it provide any evidence to support its claims about the efficacy of DL-based automatic segmentation techniques. Additionally, there is no discussion about possible risks associated with using these techniques or how they might be mitigated. Furthermore, there is no mention of any promotional content or partiality in the article, suggesting that it is unbiased and trustworthy overall.