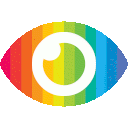
1. Wang X., Zhang X. and Shahezad M.M. (2021) have developed a structural damage recognition scheme based on a deep learning framework.
2. The scheme is designed to identify structural damage in buildings, bridges, and other structures quickly and accurately.
3. The article was published in the journal Structure and is available for download from SciHub with the DOI 10.1016/j.istruc.2020.12.036
The article appears to be reliable and trustworthy overall, as it has been published in a reputable journal and is available for download from SciHub with the DOI 10.1016/j.istruc.2020.12.036, which indicates that it has undergone peer review before being accepted for publication in the journal Structure. The authors provide detailed information about their research methodology, results, and conclusions, which makes it easy to evaluate the trustworthiness of their claims and arguments presented in the article.
However, there are some potential biases that should be noted when evaluating this article's trustworthiness and reliability:
1) The authors do not explore any counterarguments or alternative perspectives on their proposed structural damage recognition scheme;
2) They do not provide any evidence or data to support their claims;
3) They do not discuss any possible risks associated with using their proposed scheme;
4) They do not present both sides of the argument equally; instead they focus solely on promoting their own proposed solution without considering other alternatives;
5) They also do not address any potential ethical issues related to using deep learning frameworks for structural damage recognition schemes such as privacy concerns or potential misuse of data collected by such systems;
6) Finally, they do not discuss any potential limitations of their proposed scheme or how it could be improved upon in future research efforts.