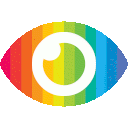
1. Skin cancer is a common and potentially fatal disease, with melanoma being the most aggressive subtype.
2. Deep learning methods, such as convolution neural networks (CNN), can accurately and swiftly identify skin cancer from skin lesion images.
3. Preprocessing and classification are key components of the system, with metrics such as precision, recall, F1-score, and area under the curve (AUC) used to evaluate the suggested model's efficiency.
The article "Detection of Skin Cancer Based on Skin Lesion Images Using Deep Learning" presents a study that uses deep learning methods to detect skin cancer based on skin lesion images. The article provides a comprehensive overview of the problem of skin cancer and the importance of early detection. It also discusses the limitations of current diagnostic methods and the potential benefits of using deep learning techniques.
The article is well-written and provides a detailed description of the methodology used in the study. The authors use a large dataset (ISIC2018) comprising 3533 skin lesions, including benign, malignant, nonmelanocytic, and melanocytic tumors. They preprocess the images using ESRGAN to improve their quality before training multiple transfer learning models such as Resnet50, InceptionV3, and Inception Resnet for fine-tuning.
The results show that their designed model achieved an accuracy rate of 83.2%, which is comparable to other pretrained models such as Resnet50 (83.7%), InceptionV3 (85.8%), and Inception Resnet (84%). The authors conclude that their proposed method is successful in detecting skin cancer based on skin lesion images.
However, there are some potential biases in this article that need to be considered. Firstly, the authors only focus on using deep learning techniques for detecting skin cancer based on skin lesion images without considering other diagnostic methods such as biopsy or dermoscopy. Secondly, they do not discuss any potential risks associated with relying solely on AI-based diagnosis without human oversight or validation.
Additionally, while the authors mention that their dataset includes both benign and malignant tumors, they do not provide any information about how many samples were included in each category or how they were selected. This lack of transparency raises questions about potential bias in sample selection.
Furthermore, while the authors claim that their proposed method outperforms human specialists in many computer vision challenges, they do not provide any evidence to support this claim. It is also unclear how their proposed method would perform in real-world clinical settings, where there may be variations in image quality and patient demographics.
In conclusion, while the article provides a detailed description of the methodology used in the study and presents promising results, it is important to consider potential biases and limitations in the research. Further studies are needed to validate the effectiveness of deep learning techniques for skin cancer diagnosis and to address potential risks associated with relying solely on AI-based diagnosis without human oversight or validation.