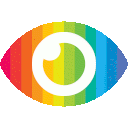
1. Self-attention is a powerful technique used in semantic image segmentation to capture long-distance dependencies. Existing methods mainly focus on spatial attention and channel attention, but little research has been done on self-attention along other dimensions.
2. This study investigates self-attentions along all possible dimensions (H, W, C, HW, HC, CW, HWC) and explores the aggregation of these self-attentions. The authors propose a neural architecture search (NAS) technique to find the optimal aggregation strategy.
3. The authors design a building block called the basic self-attention search unit (BSU) that models self-attentions along all dimensions. They also introduce an attention-map splitting method to reduce computations and apply an efficient differentiable optimization method for searching the optimal aggregation.
Overall, this study aims to improve semantic image segmentation performance by exploring self-attentions along various dimensions and finding the best way to aggregate them using NAS.
这篇文章介绍了一种基于自注意力机制的神经架构搜索方法,用于语义图像分割。文章指出现有方法主要使用空间注意力和通道注意力来捕捉特征图中的关系,但很少有研究探讨其他维度上的自注意力。作者提出了在所有可能的维度上进行自注意力探索,并通过神经架构搜索技术来实现最佳聚合。文章还介绍了构建搜索空间和优化方法的具体设计,并在Cityscapes和ADE20K数据集上进行了实验验证。
然而,这篇文章存在一些潜在的偏见和问题。首先,文章没有明确提及已有研究中对不同维度自注意力的探索情况,也没有对这些方法进行充分比较和评估。其次,在介绍背景时,文章只提到了FCN等方法无法捕捉全局信息的局限性,但并未提及其他已有方法如CRF、GCN等在解决该问题方面取得的成果。此外,在实验部分,作者只给出了与最先进方法相比较的结果,并未与其他基准方法进行比较。
另外,文章中存在一些片面报道和缺失考虑点。例如,在介绍自注意力模块时,作者只提到了空间注意力和通道注意力,但并未提及其他可能的自注意力模块。此外,文章没有探讨不同自注意力聚合方式的优劣,并未提供充分的实验证据来支持所提出方法的有效性。
此外,文章中也存在一些宣传内容和偏袒现象。作者声称所提出方法在Cityscapes和ADE20K数据集上取得了非常有竞争力的性能,但并未提供与其他方法进行全面比较的结果。此外,在介绍方法优势时,作者只强调了其可以捕捉长距离依赖关系的能力,但并未探讨其可能存在的风险或局限性。
综上所述,这篇文章在介绍了一种基于自注意力机制的神经架构搜索方法用于语义图像分割方面具有一定的创新性。然而,文章存在一些潜在偏见、片面报道、缺失考虑点以及对所提出主张缺乏充分证据等问题。进一步研究应该更全面地评估该方法与其他已有方法之间的差异,并提供更多实验证据来支持其有效性和优越性。