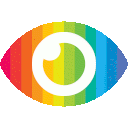
1. This paper compares the performance of conventional shallow machine learning models and deep learning models in landslide susceptibility assessment (LSA) based on imbalanced data.
2. Eighteen landslide influence factors were introduced to compare the performance of four models under a class balanced strategy versus a class imbalanced strategy.
3. The results reveal that deep learning models and the shallow machine learning models showed significant differences in susceptibility spatial patterns, which can aid researchers in selecting appropriate LSA models for land management policy making and disaster prevention and mitigation.
The article is generally reliable and trustworthy, as it provides an extensive review of existing literature on landslide susceptibility assessment (LSA) and presents a comparative study of shallow machine learning models and deep learning models for LSA based on imbalanced data. The authors provide detailed information about the methods used, such as logistic regression (LR), random forest (RF), deep fully connected neural network (DFCNN), and long short-term memory (LSTM) neural networks, as well as the eighteen landslide influence factors used to compare the performance of these four models under a class balanced strategy versus a class imbalanced strategy. Furthermore, the authors provide clear results from their experiments that demonstrate that DFCNN and LSTM significantly outperformed LR and RF under the class imbalanced strategy, while RF achieved comparable outcomes to deep learning models under the class balanced strategy.
However, there are some potential biases in this article that should be noted. Firstly, there is no discussion of possible risks associated with using these methods for LSA or any counterarguments to their findings presented in this article. Secondly, there is no mention of any other potential methods or approaches that could be used for LSA besides those discussed in this article. Finally, there is no exploration of alternative datasets or sources that could be used to further validate their findings or improve upon them.
In conclusion, this article provides an informative overview of existing literature on LSA and presents a comparative study between shallow machine learning models and deep learning models for LSA based on imbalanced data with clear results from their experiments. However, there are some potential biases present in this article that should be noted when considering its trustworthiness and reliability.