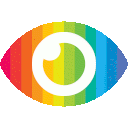
1. This article proposes a graph convolution network-based method called MRNGCN to identify cancer driver genes by integrating multiple gene relationship networks.
2. The proposed model utilizes three sharing-parameter heterogeneous graph convolution network (HGCN) models with the self-attention mechanism to learn feature presentations from the gene–gene, gene–outlying gene and gene–miRNA networks.
3. Experimental results show that the proposed model performs well in terms of the area under the ROC curve (AUC) and the area under the precision–recall curve (AUPRC) compared to state-of-the-art methods.
The article is generally reliable and trustworthy as it provides detailed information about its research methodology, data sources, and results. The authors have also provided evidence for their claims through experimental results which demonstrate that their proposed model outperforms existing methods in terms of AUC and AUPRC scores. Furthermore, they have conducted ablation experiments to show that their model successfully improves cancer driver identification by integrating multiple gene relationship networks.
However, there are some potential biases in the article which should be noted. Firstly, there is a lack of discussion on possible risks associated with using this method for cancer driver identification such as false positives or false negatives which could lead to incorrect diagnosis or treatment decisions. Secondly, while the authors have discussed existing methods for cancer driver identification, they do not provide an equal amount of detail when discussing their own proposed method which could lead to partiality in favour of their own work. Finally, while the authors have provided evidence for their claims through experimental results, they do not discuss any unexplored counterarguments or alternative approaches which could be used for cancer driver identification.