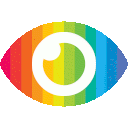
1. Meta-learning is a learning-to-learn approach that allows for rapid and accurate adaptation to unseen tasks, with applications in image classification, natural language processing, and robotics.
2. Meta-learning models can be categorized into black-box adaptation, similarity-based methods, and meta-learner procedures. Recent research has focused on combining meta-learning with Bayesian deep learning and reinforcement learning.
3. Applications of meta-learning include few-shot learning, meta-reinforcement learning, meta-imitation learning, online meta-learning, and unsupervised meta-learning. Future research directions include exploring the integration of statistical models into the meta-learning framework and developing more efficient algorithms for model adaptation.
该文章提供了对元学习的综合概述和调查,但存在一些偏见和不足之处。
首先,该文章过于强调元学习的优点,而忽略了其局限性。例如,元学习需要大量的训练数据来构建模型,并且可能会受到任务相似性假设的限制。此外,元学习方法可能会导致过拟合问题,并且在实际应用中可能会面临计算复杂度和可解释性等挑战。
其次,该文章没有充分考虑到不同领域和任务之间的差异性。例如,在自然语言处理领域,元学习方法可能会受到语言多样性和语义复杂性等因素的影响。因此,在使用元学习方法时需要更加谨慎地选择适当的模型和算法。
此外,该文章没有提供足够的证据来支持其所提出的主张。例如,在讨论贝叶斯元学习方法时,该文章没有详细说明这些方法如何改善模型泛化能力或减少过拟合问题。
最后,该文章缺少对风险和潜在问题的关注。例如,在介绍元强化学习和元模仿学习时,该文章没有探讨这些方法可能会导致的道德和伦理问题,例如机器人在执行任务时可能会对人类造成伤害。
综上所述,该文章提供了对元学习的有用概述和调查,但需要更加客观地评估其优点和局限性,并提供更多的证据来支持其主张。此外,在使用元学习方法时需要更加谨慎地考虑不同领域和任务之间的差异性,并关注潜在的风险和问题。