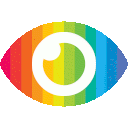
1. The methylation status of the MGMT gene is a crucial factor in determining the prognosis and treatment options for glioblastoma patients.
2. Deep learning algorithms have been proposed to predict MGMT promoter methylation from MRI scans, but there is no consensus on their effectiveness.
3. This study evaluates the performance of deep learning models for MGMT promoter methylation detection and concludes that current methods are not suitable for clinical application.
The article titled "MRI-Based Deep Learning Tools for MGMT Promoter Methylation Detection: A Thorough Evaluation" discusses the use of deep learning algorithms to predict the methylation status of the MGMT promoter in glioblastoma patients using MRI data. The authors aim to provide a comprehensive evaluation of these tools and determine their suitability for clinical application.
One potential bias in this article is the lack of discussion on the limitations and challenges associated with using deep learning algorithms for methylation prediction. While the authors acknowledge that there is no scientific consensus on this topic, they do not delve into the reasons behind this lack of consensus or address any potential issues with the current methods. This omission may give readers a skewed perspective on the effectiveness and reliability of these algorithms.
Additionally, the article does not provide a balanced view by presenting both sides of the argument regarding the use of deep learning algorithms for methylation prediction. The authors only focus on their own findings, which suggest that current methods are not suitable for clinical application. However, there may be other studies or perspectives that support the use of these algorithms. By not including these alternative viewpoints, the article presents a one-sided view of the topic.
Furthermore, there is limited evidence provided to support some of the claims made in this article. For example, when discussing previous studies on MRI-derived information and MGMT promoter status prediction, the authors mention that some studies have shown associations between certain MRI features and methylation status. However, they do not provide specific details or references to these studies, making it difficult to evaluate their validity.
The article also lacks exploration of counterarguments or alternative explanations for their findings. The authors conclude that current deep learning methods are unable to determine MGMT promoter methylation from MRI data based on their own experiments. However, they do not consider other factors that may contribute to this inability, such as sample size or variability in imaging protocols.
Additionally, there is no mention of potential risks or limitations associated with using deep learning algorithms for clinical decision-making. It is important to consider the potential for false positives or false negatives, as well as the ethical implications of relying solely on algorithmic predictions without human oversight.
Overall, this article presents a limited and biased view of the topic, with unsupported claims and missing evidence. It would benefit from a more balanced discussion of the challenges and limitations associated with using deep learning algorithms for methylation prediction, as well as a consideration of alternative viewpoints and potential risks.