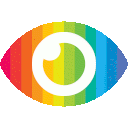
1. Materials science struggles to effectively utilize the abundance of available data, especially in applied disciplines where materials are evaluated based on device performance rather than their properties.
2. A novel NLP task called structured information inference (SII) is introduced to address the complexities of information extraction at the device level in material science, leveraging pre-existing review paper datasets.
3. Large language models (LLMs) can autonomously learn complex knowledge data frames and construct output as predefined schema from review papers without additional manual annotation, enabling direct utilization as input in subsequent data analysis without processing steps.
本文介绍了一种新的自然语言处理(NLP)任务,名为结构化信息推理(SII),旨在解决材料科学中从设备层面提取信息的复杂性。作者使用GPT-3对现有的钙钛矿太阳能电池FAIR数据集进行微调,并扩展了该数据集以包含其发布后的数据。结果表明,该方法可以高效地提取相关信息,并且生成的数据格式化和规范化,可以直接用作后续数据分析的输入。此外,作者还设计了实验来预测太阳能电池的电气性能,并使用大型语言模型(LLMs)设计具有目标参数的材料或器件。文章认为这种方法具有潜力获取科学知识并设计新材料。
然而,本文存在一些问题。首先,文章没有充分考虑到人工智能在材料科学中可能带来的风险和挑战。例如,在新材料开发过程中,可能会出现不可预见的副作用和环境影响。其次,文章没有探讨如何解决由于人工智能算法偏向某些特定领域或群体而导致的偏见问题。此外,文章未提及如何确保所使用的数据集是公正、准确和全面的,以避免数据偏差和误导性结论。最后,文章没有探讨如何平衡人工智能和人类专家的角色,以确保科学研究的可靠性和可持续性。
总之,本文提出了一种有前途的方法来解决材料科学中信息提取的复杂性,并展示了大型语言模型在科学知识获取和新材料设计方面的潜力。然而,作者需要更全面地考虑人工智能可能带来的风险和挑战,并确保所使用的数据集是公正、准确和全面的。此外,需要平衡人工智能和人类专家的角色,以确保科学研究的可靠性和可持续性。