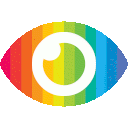
1. Current artificial intelligence (AI) models in oncology mainly operate within a single modality, limiting their potential. Integrating different data modalities can increase the accuracy and robustness of diagnostic and prognostic models.
2. AI models have the ability to discover novel patterns within and across modalities, which can contribute to the identification of biomarkers and therapeutic targets. This can guide exploration studies and improve personalized medicine.
3. Challenges in clinical adoption of AI in oncology include interpretability, scalability, and data integration. However, emerging solutions are being developed to address these challenges and facilitate the widespread use of AI in precision oncology.
The article titled "Artificial intelligence for multimodal data integration in oncology" provides an overview of the potential applications of artificial intelligence (AI) in integrating different data modalities in oncology. While the article presents valuable information on the benefits and challenges of using AI for multimodal data fusion, there are several points that need to be critically analyzed.
One potential bias in the article is its focus on the positive aspects of AI integration without adequately addressing the limitations and risks associated with this approach. The article highlights how AI models can increase the robustness and accuracy of diagnostic and prognostic models, discover novel patterns, guide exploration studies, and contribute to the discovery of biomarkers and therapeutic targets. However, it fails to mention potential issues such as data privacy concerns, algorithmic biases, interpretability challenges, and ethical considerations.
Another point to consider is the lack of evidence provided to support some of the claims made in the article. For example, it states that AI models are capable of discovering novel patterns within and across modalities suitable for explaining differences in patient outcomes or treatment resistance. While this may be true to some extent, no specific examples or studies are cited to back up this claim.
Additionally, there is a lack of discussion on potential counterarguments or alternative approaches to multimodal data integration in oncology. The article primarily focuses on AI methods but does not explore other strategies or techniques that may also be effective in integrating different data modalities.
Furthermore, there is a promotional tone throughout the article that emphasizes the benefits and potentials of AI integration without providing a balanced view. It does not adequately address potential risks or limitations associated with relying solely on AI models for decision-making in clinical practice.
Overall, while the article provides a useful overview of AI methods for multimodal data integration in oncology, it lacks critical analysis and fails to address important considerations such as limitations, risks, alternative approaches, and ethical implications. A more balanced and evidence-based discussion would have provided a more comprehensive understanding of the topic.