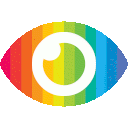
1. NiMnSn-based ferromagnetic shape memory alloys (FSMAs) have unique functional properties and are used in various applications such as magnetic refrigerators, aerospace, actuators, and sensors.
2. The martensitic transformation temperature (TM) is a crucial factor in determining the working temperature range of FSMAs. TM can be influenced by chemical composition and substitution of elements.
3. Machine learning (ML) models can be used to predict the TM of NiMnSn-based FSMAs. In this study, an ML model using the XGBRegressor algorithm was developed and showed high accuracy in predicting TM within 10 K.
The article titled "Machine-learning model for prediction of martensitic transformation temperature in NiMnSn-based ferromagnetic shape memory alloys" discusses the application of machine learning (ML) in predicting the martensitic transformation temperature (TM) of NiMnSn-based ferromagnetic shape memory alloys (FSMAs). The authors highlight the importance of TM in determining the working temperature range and suitability of FSMAs for different applications. They also mention that chemical composition plays a significant role in determining TM, and previous research has focused on adjusting the ratio of elements or substituting them to tune the thermal characteristics.
The authors argue that current methods for predicting TM, such as trial-and-error and first-principles calculations, are limited in their ability to explore the high-dimensional composition space of FSMAs due to their high cost, time consumption, or low accuracy. They propose using ML as a data-driven approach to predict TM more efficiently and accurately.
The methodology section outlines the research process, including data collection and cleaning, feature selection and engineering, model selection and training, and model verification and prediction. The authors use 127 groups of TM data for training and testing ML models. They select an optimal combination of features based on chemical composition and physical characteristics. Four ML algorithms (Random Forest Regressor, Support Vector Regression, Linear Regression, and XGBRegressor) are constructed, with XGBRegressor showing the best fitting effect.
Overall, the article provides a comprehensive overview of using ML to predict TM in NiMnSn-based FSMAs. It highlights the potential benefits of ML in accelerating research in this field. However, there are several points that need further analysis:
1. Biases: The article does not explicitly mention any biases or potential limitations associated with using ML for predicting TM. It would be helpful to discuss any inherent biases or limitations in the dataset used or potential biases introduced by the ML algorithms themselves.
2. Unsupported claims: The article claims that the XGBR model has the best fitting effect and is suitable for predicting the TM of unknown FSMAs. However, there is no detailed analysis or evidence provided to support this claim. It would be beneficial to include a comparison of the performance of different ML models and provide statistical measures such as accuracy, precision, and recall.
3. Missing evidence: The article does not provide any evidence or references to support the statement that ML has not been applied to the study of TM in NiMnSn-based FSMAs before. It would be helpful to include relevant studies or research that have explored ML applications in this field.
4. Unexplored counterarguments: The article does not discuss any potential limitations or challenges associated with using ML for predicting TM. It would be valuable to address potential issues such as overfitting, generalizability to different datasets, and interpretability of ML models.
5. Partiality: The article focuses solely on the application of ML in predicting TM and does not discuss other factors that may influence the functional properties of FSMAs, such as microstructure, processing techniques, or external conditions. A more comprehensive analysis considering these factors would provide a more holistic understanding of FSMAs.
In conclusion, while the article provides an interesting application of ML in predicting TM in NiMnSn-based FSMAs, it lacks critical analysis and supporting evidence for its claims. Further exploration of biases, limitations, counterarguments, and additional factors influencing FSMAs' functional properties would enhance the credibility and completeness of the study.